gScoreCAM: What objects is CLIP looking at?
Peijie Chen, Qi Li, Saad Biaz, Trung Bui, Anh Nguyen
Links: pdf | code | project page
Large-scale, multimodal models trained on web data such as OpenAI’s CLIP are becoming the foundation of many applications. Yet, they are also more complex to understand, test, and align with human values. In this paper, we propose gScoreCAM—a state-of-the-art method for visualizing the main objects that CLIP looks at in an image. On zero-shot object detection, gScoreCAM performs similarly to ScoreCAM, the best prior art on CLIP, yet 8 to 10 times faster. Our method outperforms other existing, well-known methods (HilaCAM, RISE, and the entire CAM family) by a large margin, especially in multiobject scenes. gScoreCAM sub-samples k = 300 channels (from 3,072 channels—i.e. reducing complexity by almost 10 times) of the highest gradients and linearly combines them into a final “attention” visualization. We demonstrate the utility and superiority of our method on three datasets: ImageNet, COCO, and PartImageNet. Our work opens up interesting future directions in understanding and de-biasing CLIP.
Acknowledgment: This work is supported by the National Science Foundation under Grant No. 1850117 & 2145767 and donations from Adobe Research and NaphCare foundation.
Conference: Asian Conference on Computer Vision (ACCV 2022). Oral presentation 6-min video | slide deck (acceptance rate: 41/836 = 4.9%)
- ⭐️ An interactive demo created by Replicate.com just for our work ⭐️
- A Google Colab demo by Peijie Chen

Figure 1: While Goh et al. 2021 at OpenAI reported that CLIP is easily fooled by typographic attacks, our gScoreCAM visualizations reveal interesting insights that CLIP indeed was able to distinguish the objects between apple, iPod and even the background. The misclassification was merely due to the fact that there are multiple objects in the scene (i.e., ill-posed, single-label, image classification task).
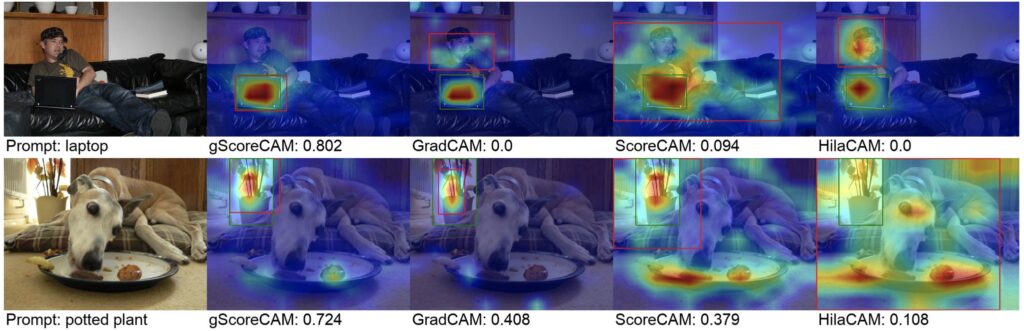
Figure 2: In complex scenes (i.e. not ImageNet-v2), gScoreCAM outperforms other methods, yielding more precise localization and cleaner heatmaps. IoU scores between the ground truth (□) and inferred box (□) are shown next to each method name.
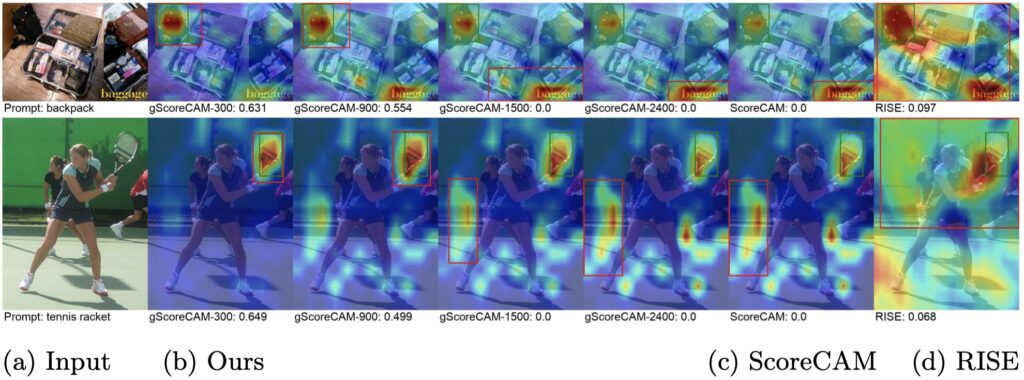
Figure 1: In a complex COCO scene, state-of-the-art feature importance methods often produce noisy heatmaps for CLIP RN50x16, questioning what objects are the most important to CLIP. Here, RISE [24] heatmap covers both suitcases and the “baggage” text (top row) while ScoreCAM [27] highlights both the racket and the tennis court (bottom row), yielding a poor 0.0 IoU between the highlighted region □ and the ground truth box □. By using only the top-300 channels of the highest gradients, we (1) localize the most important objects in a complex scene (e.g., here, racket at IoU of 0.649); and (2) produce a SotA zero-shot, openvocabulary, object localization method for COCO and PartImageNet.