Automatically identifying, counting, and describing wild animals in camera-trap images with deep learning
Mohammad S. Norouzzadeh, Anh Nguyen, Margaret Kosmala, Alexandra Swanson, Meredith S. Palmer, Craig Packer, and Jeff Clune
Links: pdf | code | project page
Motion-sensor cameras in natural habitats offer the opportunity to inexpensively and unobtrusively gather vast amounts of data on animals in the wild. A key obstacle to harnessing their potential is the great cost of having humans analyze each image. Here, we demonstrate that a cutting-edge type of artificial intelligence called deep neural networks can automatically extract such invaluable information. For example, we show deep learning can automate animal identification for 99.3% of the 3.2 million-image Snapshot Serengeti dataset while performing at the same 96.6% accuracy of crowdsourced teams of human volunteers. Automatically, accurately, and inexpensively collecting such data could help catalyze the transformation of many fields of ecology, wildlife biology, zoology, conservation biology, and animal behavior into “big data” sciences.
Journal: Proceedings of the National Academy of Sciences (PNAS) June 19, 2018 115 (25) E5716-E5725; first published June 5, 2018 https://doi.org/10.1073/pnas.1719367115
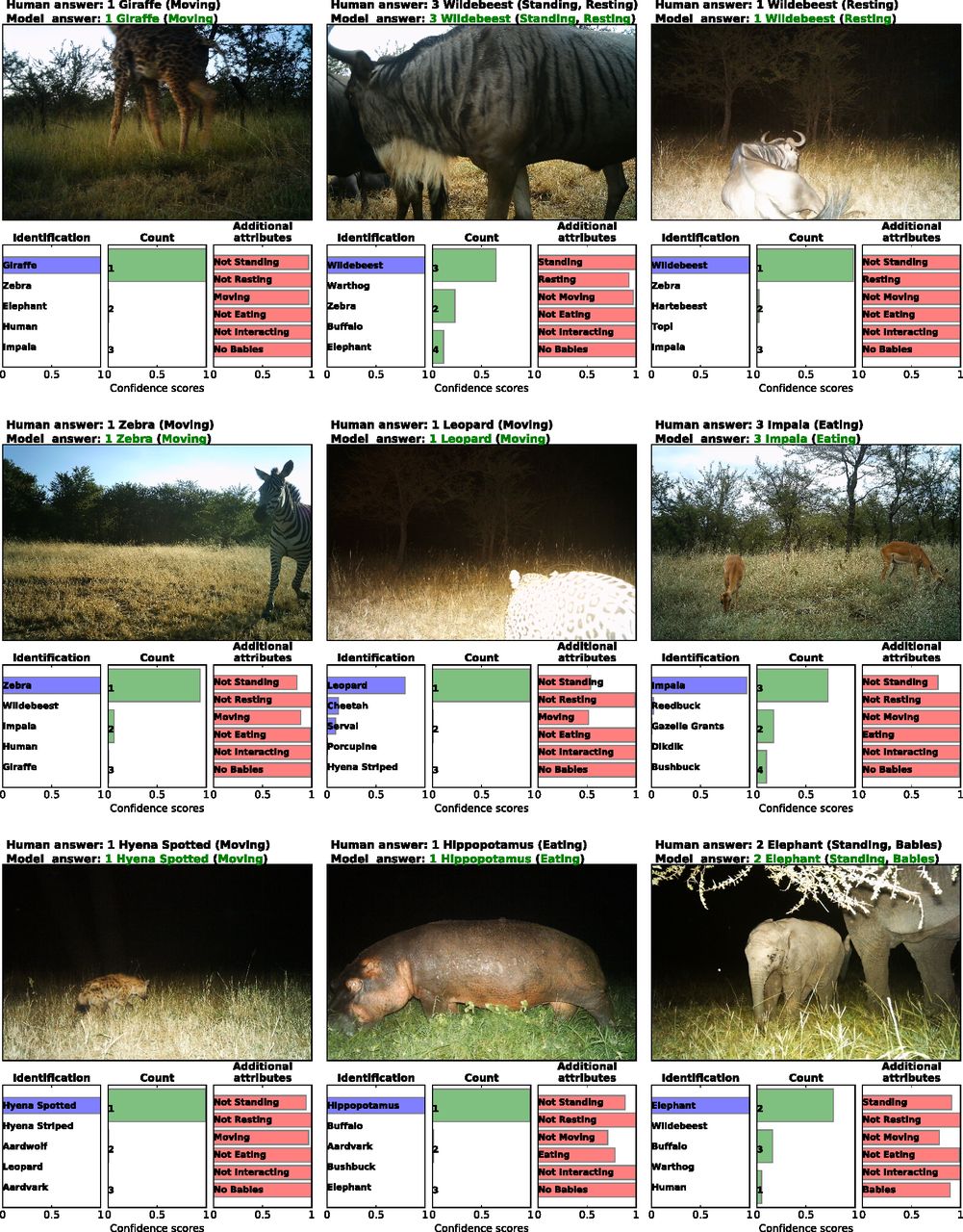
Shown are nine images the ResNet-152 model labeled correctly. Above each image is a combination of expert-provided labels (for the species type and counts) and volunteer-provided labels (for additional attributes), as well as the model’s prediction for that image. Below each image are the top guesses of the model for different tasks, with the width of the color bars indicating the model’s output for each of the guesses, which can be interpreted as its confidence in that guess. Deep neural networks (DNNs) can successfully identify, count, and describe animals in camera-trap images. Above the image: The ground-truth, human-provided answer (top line) and the prediction (second line) by a DNN we trained (ResNet-152). The three plots below the image, from left to right, show the neural network’s prediction for the species, number, and behavior of the animals in the image. The horizontal color bars indicate how confident the neural network is about its predictions. All similar images in this work are from the SS dataset.